Rerender A Video: Zero-Shot Text-Guided Video-to-Video Translation
Paper
Abstract
Large text-to-image diffusion models have exhibited impressive proficiency in generating high-quality images. However, when applying these models to video domain, ensuring temporal consistency across video frames remains a formidable challenge. This paper proposes a novel zero-shot text-guided video-to-video translation framework to adapt image models to videos. The framework includes two parts: key frame translation and full video translation. The first part uses an adapted diffusion model to generate key frames, with hierarchical cross-frame constraints applied to enforce coherence in shapes, textures and colors. The second part propagates the key frames to other frames with temporal-aware patch matching and frame blending. Our framework achieves global style and local texture temporal consistency at a low cost (without re-training or optimization). The adaptation is compatible with existing image diffusion techniques, allowing our framework to take advantage of them, such as customizing a specific subject with LoRA, and introducing extra spatial guidance with ControlNet. Extensive experimental results demonstrate the effectiveness of our proposed framework over existing methods in rendering high-quality and temporally-coherent videos.
* Web demo:
* Full code: According to the Anonymity Policy, we will release the full code and data upon the publication of the paper.
The
Hierarchical Cross-Frame Constraints
We propose novel hierarchical cross-frame constraints for pre-trained image diffusion models to produce coherent video frames. Our key idea is to use optical flow to apply dense cross-frame constraints, with the previous rendered frame serving as a low-level reference for the current frame and the first rendered frame acting as an anchor to regulate the rendering process to prevent deviations from the initial appearance. Hierarchical cross-frame constraints are realized at different stages of diffusion sampling. In addition to global style consistency (cross-frame attention), our method enforces consistency in shapes (shape-aware cross-frame latent fusion), textures (pixel-aware cross-frame latent fusion) and colors (color-aware adaptive latent adjustment) at early, middle and late stages, respectively. This innovative and lightweight modification achieves both global and local temporal consistency.
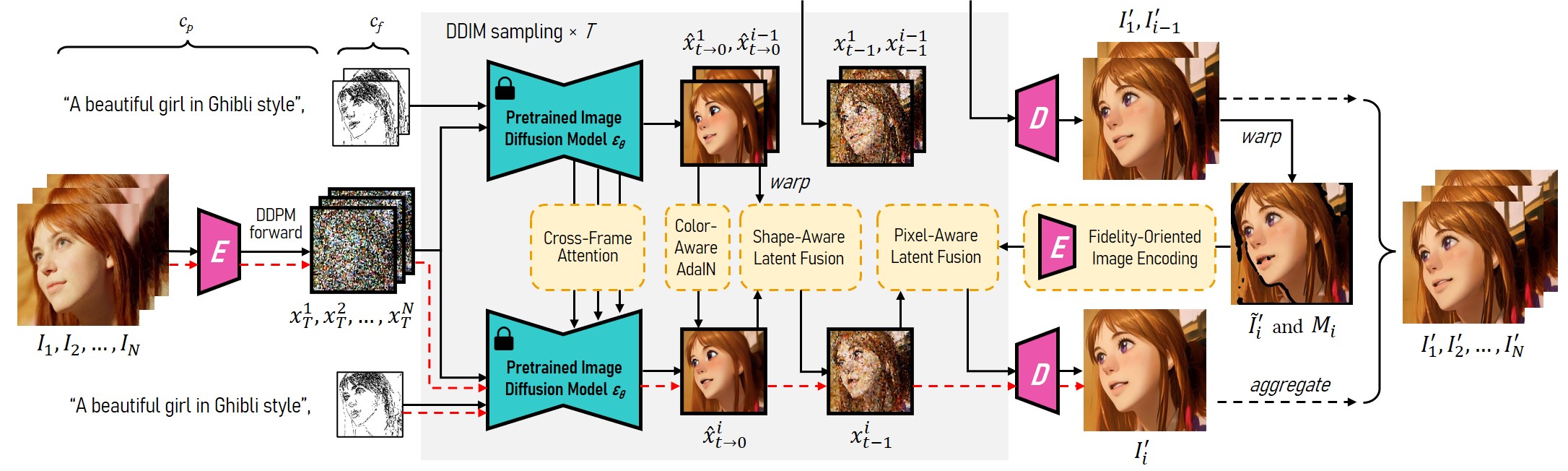
Effect of
Temporal Consistency Constraints
Our framework explores several temporal consistency constraints to generate smooth results. Their role is studied below. Move the mouse (computer) or double-click (phone) over the video to make a better comparison.
Left: Original image model (Stable Diffusion). Right: Our adapted video model.
Experimental
Results
Comparison with zero-shot text-guided video translation methods
We compare with four recent zero-shot methods: vid2vid-zero, FateZero, Pix2Video, Text2Video-Zero. FateZero successfully reconstructs the input frame but fails to adjust it to match the prompt. On the other hand, vid2vid-zero and Pix2Video excessively modify the input frame, leading to significant shape distortion and discontinuity across frames. While each frame generated by FateZero exhibits high quality, they lack coherence in local textures. Finally, our proposed method demonstrates clear superiority in terms of output quality, content and prompt matching and temporal consistency.